Can Machine Learning Predict And Prevent Fraudsters?
How Machine Learning Systems Detect And Prevent Frauds Without Affecting Your Customers
There is nothing more fearful than imbalanced data, especially when dealing with various payment channels like credit and debit cards in banks and other financial organizations. With the wide increase of different payment mediums, businesses are finding it difficult to authenticate transactions. But Machine Learning has been a viable solution to detect fraudsters.
Machine Learning can be referred to as the ability of machines to learn data with the help of human intelligence as well. According to the latest report by Gartner, by 2022, more than nearly half the data and analytics services/ tasks will be done by machines.
Related Reading: Read on to learn how machine learning can help boost customer experience.
Machine Learning In Making Real-Time Decisions To Prevent Fraud Activities
If a business is able to predict which transactions can lead to fraudster attacks, then the business can considerably lower costs and make critical decisions. While sending sensitive data to a third-party, it is important that the data is not misused for fraudulent activities. This can be done as follows:
-
Using Machine Learning Models
Consider a score produced from a number of algorithms that is a combination of all possible features. This set of algorithms can be termed as a machine learning model. This machine learning model constantly queries these algorithms in order to produce an accurate score that can be used to predict frauds.
Machine learning models can be compared to data analysts who run numerous queries on large volumes of data and try finding out the best from the derived outcomes. Machine Learning makes the whole process fast and accurate.
-
Fraud Scores For Fraud Detection
There always exists large amounts of data. Machines are trained using these data sets that are pre-labeled as frauds. These labels are based on earlier records of confirmed fraudulent activities.
The machines are then trained using this labeled set of data. These data sets are now called as training sets. By a named label, the machine is taught to determine if a new transaction or a particular customer is likely to be a fraudster based on a score of 0 to 100, being the probability.
This score enhances the ability of a business to ensure a considerable reduction in frauds by providing accurate predictions.
Related Reading: Check on to this Infographic to learn more about Machine Learning.
Can Machine Learning Actually Predict And Prevent Fraudsters?
Designing as well as being able to apply algorithms that are on the basis of data sets from the past, enables to analyze frequent patterns in these data sets. These patterns in data via the algorithm are taught to machines and these machines considerably reduce human effort.
These algorithms help businesses boost predictive analysis. Predictive analysis is important for data reduction by using statistical modeling techniques that help in predicting future business outcomes on the basis of past data patterns. In fact, among many businesses, 75 percent of them find growth to be their main source of value, whereas 60 percent of some others believe that it is nothing else but predictive analytics that is the key to deriving value!
Machine learning algorithms are not only used in predictive analytics, but also in image recognition, detecting spam, and so on. Machine Learning can be trained by a 3 phase system.
1. Train
2. Test
3. Predict
So to be able to predict an occurrence of fraud in large volumes of data sets and transactions, cognitive technologies of computing are applied to raw and unprocessed data.
Machine Learning thus facilitates, prediction and prevention of fraudsters for the following key factors:
- Scalability: Larger the data sets, increased is the effectiveness of machine learning algorithms. Initially, the machine learns which transaction/data sets are fraudulent and which ones are safe, the machines are well able to predict such cases in future transactions.
- Readiness: Manual tasks are time-consuming. These are not preferred by clients. Hence, machine learning strategies are used to acquire faster results. Machine learning algorithms process a large number of data sets in real-time to customers. Machine Learning frequently and periodically analyzes and processes new data sets. Advanced models like neural networks have provisions for autonomous updations in real-time.
- Productivity: The need to perform redundant tasks reduces productivity. The continuous repetitive task of data analysis is performed by Machine Learning algorithms and prompts for human intervention only when required.
Related Reading: Check out how machine learning is revolutionizing software development.
Machine Learning Methods – Using White Boxes And Ongoing Monitoring To Detect Fraudsters
What does a machine learning system do? The methods adopted and the various approaches used for this are termed Whiteboxes, as there is no definite method or model to analyze the score obtained. Similarly, regular and ongoing monitoring is critical for a machine learning system to identify the trends and data statistics on a regular basis.
How Fraudsters Are Detected And Prevented By Using Machine Learning
Data sets are initially collected and partitioned. The machine learning model is taught the sets in order to predict data fraud. The following are the steps in which Machine Learning implements and performs fraud detection:
- Data Partitioning: The data is segmented into working in three different phases such as training the machine, testing for data sets and finally, cross-checking of the prediction results.
- Obtaining Results of Historical Data: To obtain such data sets, training sets have to be first provided to the machine that includes input values associated with its corresponding output values. This helps in predicting and detecting frauds.
- Predicting Anomalies, If Any: Based on the input and output data, predictions are determined by analyzing the anomalies or fraud cases in the data sets. For this, building models are used. This can be done by many techniques such as using Decision Trees, Logistic Regression, Neural Networks, and Random Forests, etc.
- Out of the techniques, Neural Networks are quick in processing results by analyzing data sets and helps in making decisions in real-time. It does so by observing regular patterns of frauds in earlier cases of data sets given to it for learning.
In a nutshell, Machine Learning is proving to be the right technology in detecting and preventing fraudsters from malicious activities. If banks start using machine learning systems, it could analyze unstructured data and prevent customer’s accounts from fraudulent activities. To know more about how you can empower machine learning and other technology trends to secure data, get in touch with our IT experts today!
Stay up to date on what's new
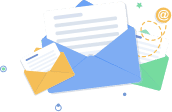
Recommended Posts
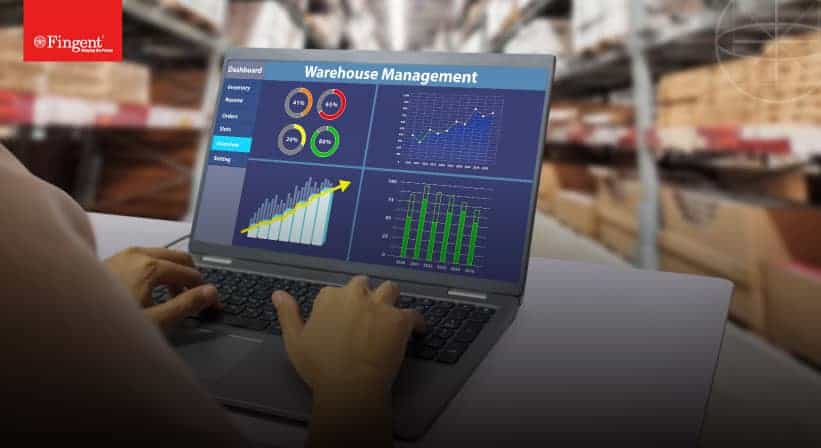
25 Jun 2024 Financial Services B2B
Business Intelligence in Financial Services: Unlocking Data-Driven Success
Business Intelligence in Financial Services is proving to be a game changer. Business intelligence is a novel technology backed by AI. It is a combination of strategies and processes. Simply……
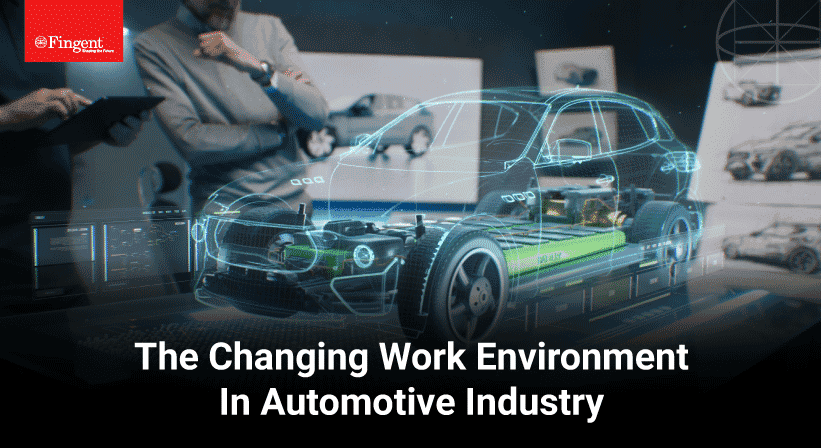
10 Mar 2023 B2B
Looking Ahead: The Future of Work in the Automotive Industry!
The automobile industry is one of the largest sectors in the world. In just the U.S., the car & automobile manufacturing industry boasts a market size of $104.1 billion. However,……
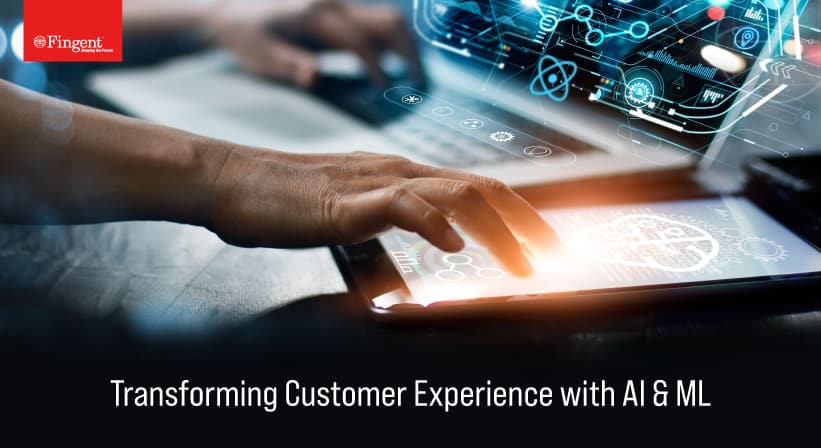
14 Feb 2023 B2B
How AI and ML Are Reshaping Customer Experiences
No longer the stuff of science fiction, artificial intelligence (AI) and machine learning (ML) are revolutionizing the way customers interact with brands. Businesses that have embraced these technologies can reshape……
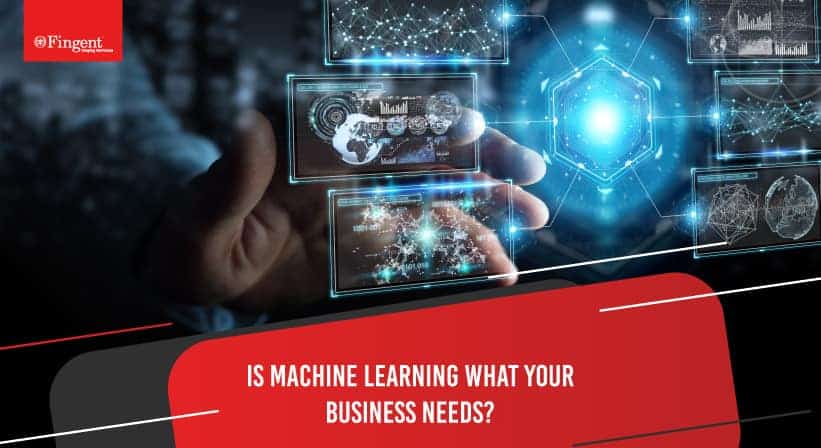
10 Nov 2022 B2B
Machine Learning in Business: Use Cases & Business Benefits
Machine learning is changing the face of everyday life, science, and business. It is revolutionizing all industries, from advancing medicine to powering various cutting-edge technologies. Though Machine learning (ML) was……
Featured Blogs
Stay up to date on
what's new
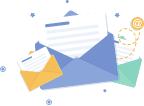
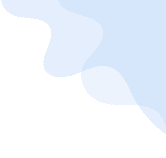
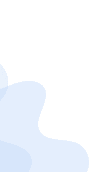